See also
A Jupyter notebook version of this tutorial can be downloaded here
.
Rabi experiment
In this tutorial we will combine the techniques explained in the other tutorials and show how to perform a Rabi experiment. For this tutorial we will need one QCM to generate the Rabi pulses and one QRM to perform the readout, although the QCM could be replaced by another QRM if needed.
Ports \(\text{O}^{[1-2]}\) of the QCM are used for the driving pulse, while \(\text{O}^{[1-2]}\) of the QRM are used for the readout pulse. Finally, ports \(\text{I}^{[1-2]}\) are used for the acquisition of the readout tone. In this tutorial it is assumed \(\text{O}^{1}\) of the QRM is connected to \(\text{I}^{1}\) of the QRM for time of flight calibration. Furthermore we assume that \(\text{O}^{1}\) of the QCM and \(\text{O}^{2}\) of the QRM are connected to an external oscilloscope to view the Rabi experiment pattern. The scope can be triggered of marker 1 of the QCM.
As demonstrated in the synchronization tutorial, the SYNQ technology synchronizes the programs in the two modules. If you are using Pulsar modules, to ensure synchronization between the modules, connect the \(\text{REF}^{out}\) of the Pulsar QCM to the \(\text{REF}^{in}\) of the Pulsar QRM using a coaxial cable, and connect their SYNQ ports using the SYNQ cable.
If you are using a Cluster, then no extra connection needs to be made as the modules are internally connected with SYNQ capability.
To run this tutorial please make sure you have installed and enabled ipywidgets:
pip install ipywidgets
jupyter nbextension enable --py widgetsnbextension
Setup
First, we are going to import the required packages and connect to the instrument.
[1]:
# Import ipython widgets
import json
import math
import ipywidgets as widgets
import matplotlib.pyplot as plt
import numpy as np
# Set up the environment.
import scipy.signal
from IPython.display import display
from ipywidgets import fixed, interact, interact_manual, interactive
from qcodes import Instrument
from qblox_instruments import Cluster, PlugAndPlay, Pulsar
Scan For Devices
We scan for the available devices connected via ethernet using the Plug & Play functionality of the Qblox Instruments package (see Plug & Play for more info).
[2]:
# Scan for available devices and display
with PlugAndPlay() as p:
# get info of all devices
device_list = p.list_devices()
names = {
dev_id: dev_info["description"]["name"] for dev_id, dev_info in device_list.items()
}
ip_addresses = {
dev_id: dev_info["identity"]["ip"] for dev_id, dev_info in device_list.items()
}
# create widget for names and ip addresses
connect = widgets.Dropdown(
options=[(names[dev_id] + " @" + ip_addresses[dev_id], dev_id)
for dev_id in device_list.keys()],
description="Select Device",
)
display(connect)
The following widget displays all the existing modules that are connected to your PC which includes the Pulsar modules as well as a Cluster. Select the device you want to run the notebook on.
Connect to Cluster
We now make a connection with the Cluster selected in the dropdown widget. We also define a function to find the modules we’re interested in. We select the readout and control module we want to use.
[ ]:
# Connect to device
dev_id = connect.value
# Close the chosen QCodes instrument as to prevent name clash.
try:
Instrument.find_instrument(names[dev_id]).close()
except KeyError:
pass
cluster = Cluster(name=names[dev_id], identifier=ip_addresses[dev_id], debug=True) # TODO remove debug
print(f"{connect.label} connected")
print(cluster.get_system_state())
[ ]:
def select_module_widget(device, select_all=False, select_qrm_type: bool=True, select_rf_type: bool=False):
"""Create a widget to select modules of a certain type
default is to show only QRM baseband
Args:
devices : Cluster we are currently using
select_all (bool): ignore filters and show all modules
select_qrm_type (bool): filter QRM/QCM
select_rf_type (bool): filter RF/baseband
"""
options = [[None, None]]
for module in device.modules:
if module.present():
if select_all or (module.is_qrm_type == select_qrm_type and module.is_rf_type == select_rf_type):
options.append(
[
f"{device.name} "
f"{module.short_name} "
f"({module.module_type}{'_RF' if module.is_rf_type else ''})",
module,
]
)
widget = widgets.Dropdown(options=options)
display(widget)
return widget
[ ]:
print("Select the readout module from the available modules:")
select_readout_module = select_module_widget(cluster, select_qrm_type=True, select_rf_type=False)
[ ]:
readout_module = select_readout_module.value
print(f"{readout_module} connected")
[ ]:
print("Select the control module from the available modules:")
select_control_module = select_module_widget(cluster, select_qrm_type=False, select_rf_type=False)
[ ]:
control_module = select_control_module.value
print(f"{control_module} connected")
Sequencer Setup
Set sync_en
to synchronize across modules.
[7]:
# Set sync_en
readout_module.sequencer0.sync_en(True)
control_module.sequencer0.sync_en(True)
Configure the NCO
of both the QRM and QCM to 100 MHz and enable the up- and down-conversion in the sequencers
[8]:
readout_module.sequencer0.nco_freq(100e6)
readout_module.sequencer0.mod_en_awg(True)
readout_module.sequencer0.demod_en_acq(True)
control_module.sequencer0.nco_freq(100e6)
control_module.sequencer0.mod_en_awg(True)
Configure the outputs of the QRM and QCM such that sequencer0 is the only enabled sequencer and maps to \(\text{O}^{[1-2]}\)
[9]:
# Map sequencer of the QCM to specific outputs (but first disable all sequencer connections)
for sequencer in control_module.sequencers:
sequencer.disconnect_outputs()
control_module.sequencer0.connect_sequencer("out0_1")
# Map sequencer of the QRM to specific outputs (but first disable all sequencer connections)
for sequencer in readout_module.sequencers:
sequencer.disconnect_outputs()
sequencer.disconnect_inputs()
readout_module.sequencer0.connect_sequencer("io0_1")
Define waveforms
To readout the systems we define constant pulses one
and zero
which will be up converted by the NCO
to create the appropriate tones for an IQ mixer. Similarly for driving the qubit, we define a Gaussian pulse, together with a zero pulse of equal length to serve as inputs for an IQ mixer. In this tutorial we do not assume mixers to be connected to the inputs and outputs of the QCM/QRM.
[10]:
t = np.arange(-80, 81, 1)
sigma = 20
wfs = {
"zero": {"index": 0, "data": [0.0] * 1024},
"one": {"index": 1, "data": [1.0] * 1024},
"gauss": {"index": 2, "data": list(np.exp(-(0.5 * t**2 / sigma**2)))},
"empty": {"index": 3, "data": list(0.0 * t)},
}
Hence we obtain the following waveforms for readout:
[11]:
plt.plot(wfs["zero"]["data"])
plt.plot(wfs["one"]["data"])
[11]:
[<matplotlib.lines.Line2D at 0x29bf811b850>]
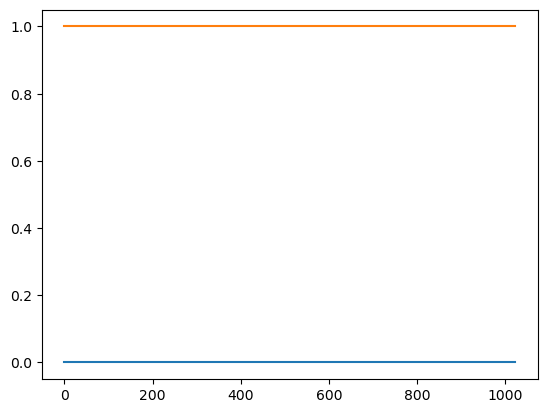
And for drive:
[12]:
plt.plot(wfs["gauss"]["data"])
plt.plot(wfs["empty"]["data"])
[12]:
[<matplotlib.lines.Line2D at 0x29bf8361bb0>]
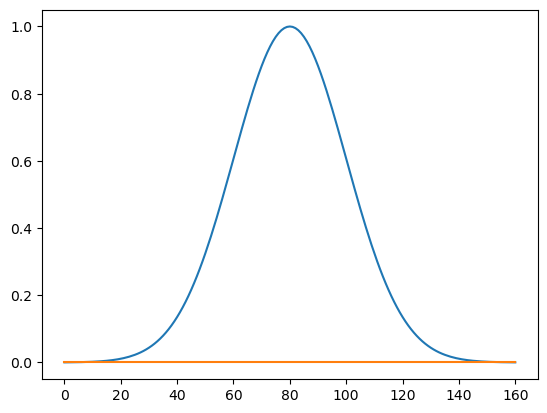
Finally, we define two acquisitions. A single readout to perform the calibration measurements. Secondly we create a rabi
readout sequence that contains 50 different bins for saving the results, one for each of the different amplitudes used for the drive tone.
[13]:
num_bins = 50 # Number of amplitudes to be measured
acquisitions = {
"single": {"num_bins": 1, "index": 0},
"rabi": {"num_bins": num_bins, "index": 1},
}
Calibration experiments
TOF calibration
As a first step, we calibrate the time of flight (tof) for the QRM module. In order to do so, we play a readout pulse and analyze the obtained signal on the oscilloscope to find the travel time of the pulse through the system.
[14]:
qrm_prog = f"""
play 1, 0, 4 # start readout pulse
acquire 0, 0, 16384 # start the 'single' acquisition sequence and wait for the length of the scope acquisition window
stop
"""
Upload the program, together with the waveforms and acquisitions to the QRM
[15]:
sequence = {
"waveforms": wfs,
"weights": {},
"acquisitions": acquisitions,
"program": qrm_prog,
}
with open("sequence.json", "w", encoding="utf-8") as file:
json.dump(sequence, file, indent=4)
file.close()
# Upload sequence.
readout_module.sequencer0.sequence("sequence.json")
Perform the calibration experiment
[16]:
# Arm and start sequencer.
readout_module.arm_sequencer(0)
readout_module.start_sequencer()
# Wait for the sequencer and acquisition to finish with a timeout period of one minute.
readout_module.get_acquisition_state(0, 1)
readout_module.store_scope_acquisition(0, "single")
# Print status of sequencer.
print(readout_module.get_sequencer_state(0))
Status: STOPPED, Flags: FORCED_STOP, ACQ_SCOPE_DONE_PATH_0, ACQ_SCOPE_DONE_PATH_1, ACQ_BINNING_DONE
Analyze the resulting signal on the scope to find the tof
[17]:
p0 = np.array(
readout_module.get_acquisitions(0)["single"]["acquisition"]["scope"]["path0"]["data"]
)
p1 = np.array(
readout_module.get_acquisitions(0)["single"]["acquisition"]["scope"]["path1"]["data"]
)
# Determine when the signal crosses half-max for the first time (in ns)
t_halfmax = np.where(np.abs(p0) > np.max(p0) / 2)[0][0]
# The time it takes for a sine wave to reach its half-max value is (in ns)
correction = 1 / readout_module.sequencer0.nco_freq() * 1e9 / 12
tof_measured = t_halfmax - correction
Plot the signal on the scope, around the rising and falling edge of the acquisition signal, as determined by the tof analysis above:
[18]:
r = readout_module.get_acquisitions(0)["single"]["acquisition"]["scope"]
plt.plot(r["path0"]["data"], ".-")
plt.plot(r["path1"]["data"], ".-")
plt.axvline(tof_measured, c="k")
plt.xlim(
tof_measured - 10 / readout_module.sequencer0.nco_freq() * 1e9,
tof_measured + 10 / readout_module.sequencer0.nco_freq() * 1e9,
)
plt.show()
plt.plot(r["path0"]["data"], ".-")
plt.plot(r["path1"]["data"], ".-")
plt.axvline(1024 + tof_measured, c="k")
plt.xlim(
1024 + tof_measured - 10 / readout_module.sequencer0.nco_freq() * 1e9,
1024 + tof_measured + 10 / readout_module.sequencer0.nco_freq() * 1e9,
)
plt.show()
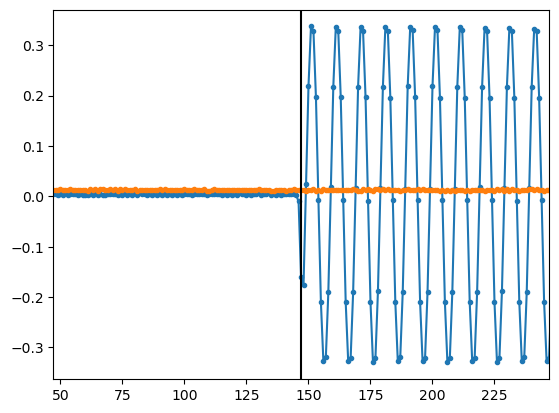
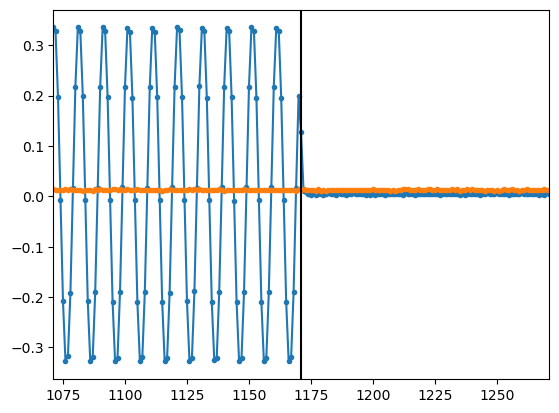
Parameters
Set the parameters for the Rabi experiment
[19]:
# all times must be divisible by 4
reset_time = 200 # reset time for the qubit in microseconds
tof = int(tof_measured / 4) * 4 # time of flight must be divisible by 4
readout_delay = (
164 # time to delay the readout pulse after the start of the rotation pulse
)
navg = 1000 # number of averages
stepsize = int(65535 / 100)
Rabi
Normally, a Rabi experiment would be performed by changing the amplitude in the inner loop, and averaging in the outer loop. To make the resulting experiment visible on an oscilloscope however, in this tutorial we swapped these two loops
[20]:
# QCM sequence program.
qcm_seq_prog = f"""
# Registers used:
# R0 loops over the different awg amplitudes used for the rabi driving pulse
# R2 is used to count the averages needed for a single amplitude
# R3 contains the qubit reset time in microseconds
move 0, R0 # start with awg amplitude 0
wait_sync 4 # Synchronize the QRM with the QCM
ampl_loop: add R0, {stepsize}, R0 # increase the pulse amplitude by the stepsize
move {navg}, R2 # reset the number of averages and save in the R2 register
# let the qubit relax to its groundstate
navg_loop: move {reset_time}, R3 # reset the number of microseconds to wait and save in the R3 register
rst_loop: wait 1000 # wait 1 microsecond
loop R3,@rst_loop # repeat the 1 microsecond wait as much as needed to let the qubit relax
set_awg_gain R0, R0 # Set the new amplitude used for the drive pulse
set_mrk 1 # Set marker 1 high for to enable synchronization with external oscilloscope
wait_sync 4 # Synchronize with the qrm to signify a measurement is coming
play 2,3,16384 # Play waveforms and wait remaining duration of scope acquisition
set_mrk 0 # Reset marker 1
upd_param 4
loop R2,@navg_loop # Repeat the experiment to average, until R2 becomes 0
jlt R0,{num_bins*stepsize},@ampl_loop # Repeat the experiment for different pulse amplitudes from 0 to num_bins
stop # Stop.
"""
# QRM sequence program.
qrm_seq_prog = f"""
# Registers used:
# R0 counts which bin to acquire into, a new bin for every new amplitude
# R2 is used to count the averages needed for a single amplitude
wait_sync 4 # Synchronize the QRM with the QCM.
move 0, R0 # the first acquisition uses bin 0
ampl_loop: move {navg}, R2 # reset the amount of averages to be taken to the initial value
navg_loop: wait_sync {readout_delay} # wait for the QCM to signal a pulse is coming and wait the readout_delay
play 1,0,{tof} # play readout pulse and wait for the tof
acquire 1,R0,16384 # Acquire waveforms and wait remaining duration of scope acquisition.
loop R2, @navg_loop # Repeat this measurement for every average
add R0,1,R0 # Increment the bin into which we are measuring
jmp @ampl_loop # repeat
"""
Upload programs and waveforms to QRM and QCM
[21]:
# Add QCM sequence to single dictionary and write to JSON file.
sequence = {
"waveforms": wfs,
"weights": {},
"acquisitions": acquisitions,
"program": qcm_seq_prog,
}
with open("qcm_sequence.json", "w", encoding="utf-8") as file:
json.dump(sequence, file, indent=4)
file.close()
# Add QRM sequence to single dictionary and write to JSON file.
sequence = {
"waveforms": wfs,
"weights": {},
"acquisitions": acquisitions,
"program": qrm_seq_prog,
}
with open("qrm_sequence.json", "w", encoding="utf-8") as file:
json.dump(sequence, file, indent=4)
file.close()
# Upload sequence to QCM.
control_module.sequencer0.sequence("qcm_sequence.json")
# Upload sequence to QRM.
readout_module.sequencer0.sequence("qrm_sequence.json")
Arm and start sequencer0 of both the QCM and QRM. The wait_sync
command together with the SYNQ technology ensures both modules start simultaneously.
[22]:
# Arm and start sequencer of the QCM (only sequencer 0).
control_module.arm_sequencer(0)
control_module.start_sequencer(0)
# Print status of sequencer of the QCM.
print("QCM:")
print(control_module.get_sequencer_state(0))
print()
# Arm and start sequencer of the QRM (only sequencer 0).
readout_module.arm_sequencer(0)
readout_module.start_sequencer(0)
# Print status of sequencer of the QRM.
print("QRM:")
print(readout_module.get_sequencer_state(0))
print("QCM:")
print(control_module.get_sequencer_state(0, 1))
readout_module.stop_sequencer(
0
) # We didn't tell the QRM how many different amplitudes would be measured, so here we tell it to stop.
print("QRM:")
print(readout_module.get_sequencer_state(0, 1))
QCM:
Status: RUNNING, Flags: NONE
QRM:
Status: RUNNING, Flags: ACQ_SCOPE_DONE_PATH_0, ACQ_SCOPE_OVERWRITTEN_PATH_0, ACQ_SCOPE_DONE_PATH_1, ACQ_SCOPE_OVERWRITTEN_PATH_1, ACQ_BINNING_DONE
QCM:
Status: STOPPED, Flags: NONE
QRM:
Status: STOPPED, Flags: FORCED_STOP, ACQ_SCOPE_DONE_PATH_0, ACQ_SCOPE_OVERWRITTEN_PATH_0, ACQ_SCOPE_DONE_PATH_1, ACQ_SCOPE_OVERWRITTEN_PATH_1, ACQ_BINNING_DONE
Stop
Finally, let’s stop the sequencers if they haven’t already and close the instrument connection. One can also display a detailed snapshot containing the instrument parameters before closing the connection by uncommenting the corresponding lines.
[23]:
# Stop sequencers.
control_module.stop_sequencer()
readout_module.stop_sequencer()
# Print status of sequencers.
print("QCM :")
print(control_module.get_sequencer_state(0))
print()
print("QRM :")
print(readout_module.get_sequencer_state(0))
print()
# Uncomment the following to print an overview of the instrument parameters.
# Print an overview of instrument parameters.
# print("QCM snapshot:")
# qcm.print_readable_snapshot(update=True)
print()
# print("QRM snapshot:")
# qrm.print_readable_snapshot(update=True)
# Close the instrument connections.
Pulsar.close_all()
Cluster.close_all()
QCM :
Status: STOPPED, Flags: FORCED_STOP
QRM :
Status: STOPPED, Flags: FORCED_STOP, ACQ_SCOPE_DONE_PATH_0, ACQ_SCOPE_OVERWRITTEN_PATH_0, ACQ_SCOPE_DONE_PATH_1, ACQ_SCOPE_OVERWRITTEN_PATH_1, ACQ_BINNING_DONE
[ ]: